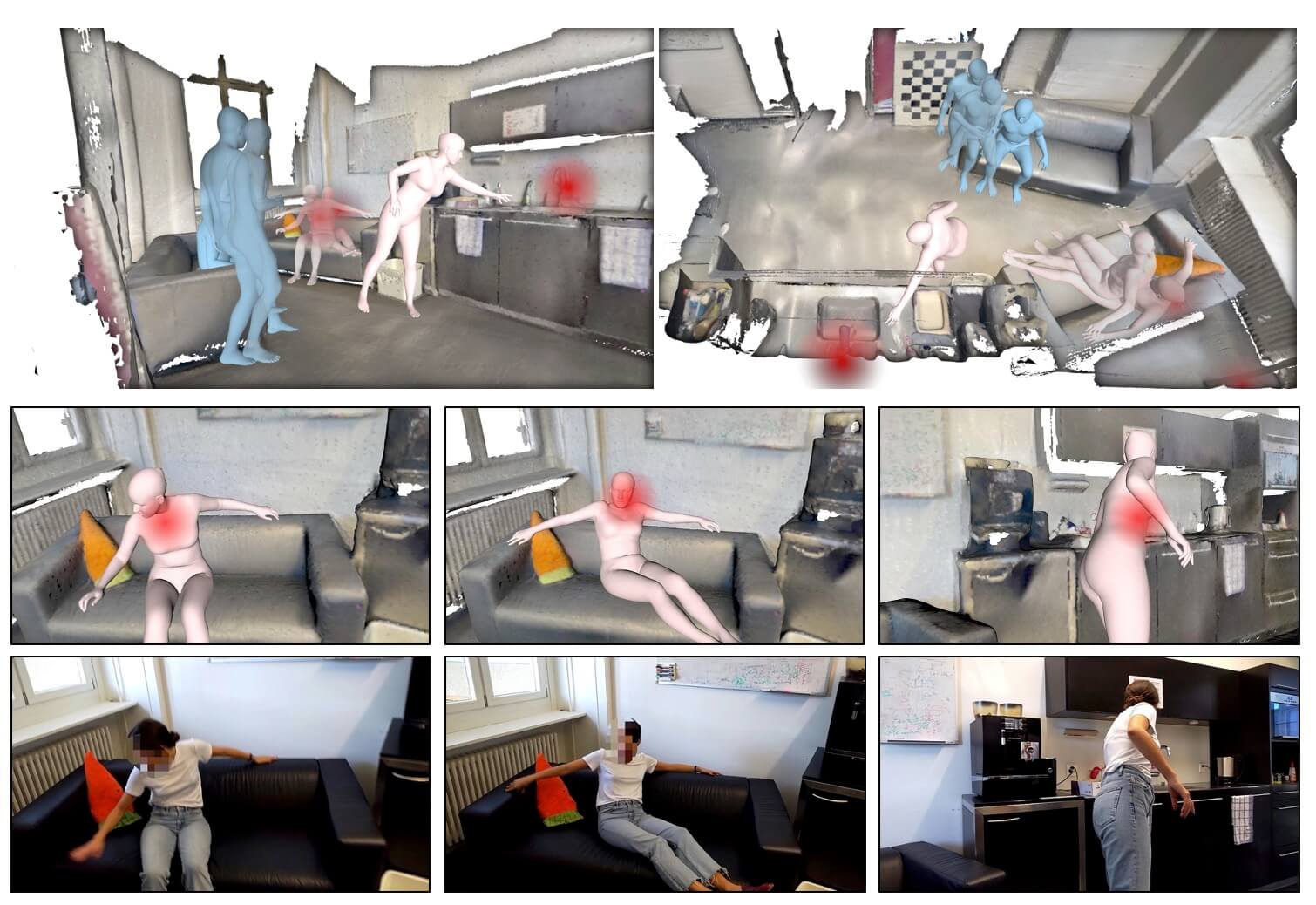
With the continuous advancement of mobile processing and the increasing availability of extended reality (XR) headsets, extended reality will soon become available to the masses. We Envision a future world where humans, objects, and scenes from the physical and digital worlds are merged. In this embodied and immersive future, the ability to understand humans is essential for extended reality headsets. However, current work in the computer vision literature mainly focuses on third-person view scenarios. The egocentric perception is a fundamentally new and challenging arena, where existing data and models learned in the third-person view setting do not generalize to the unprecedented scale and diversity of the egocentric setting. Our group has focused on bridging this gap. To understand human-human interactions from egocentric views, we have proposed novel datasets (EgoBody) and methods (4DCapture) to reconstruct human motion. Furthermore, together with Microsoft and the ETH AI center, we will host the first egocentric human pose and shape estimation challenge in conjunction with ECCV2022. By sharing our data and benchmark with the research community, we envision our work as a major milestone to unlock 3D human body estimation in the egocentric setting.
Publications
Authors:Siwei Zhang, Qianli Ma, Yan Zhang, Zhiyin Qian, Taein Kwon, Marc Pollefeys, Federica Bogo and Siyu Tang
A large-scale dataset of accurate 3D human body shape, pose and motion of humans interacting in 3D scenes, with multi-modal streams from third-person and egocentric views, captured by Azure Kinects and a HoloLens2.Authors:Miao Liu, Dexin Yang, Yan Zhang, Zhaopeng Cui, James M. Rehg, Siyu Tang
We seek to reconstruct 4D second-person human body meshes that are grounded on the 3D scene captured in an egocentric view. Our method exploits 2D observations from the entire video sequence and the 3D scene context to optimize human body models over time, and thereby leads to more accurate human motion capture and more realistic human-scene interaction.Authors:Miao Liu, Siyu Tang, Yin Li, and James M. Rehg
We address the challenging task of anticipating human-object interaction in first person videos. We adopt intentional hand movement as a future representation and propose a novel deep network that jointly models and predicts the egocentric hand motion, interaction hotspots and future action.